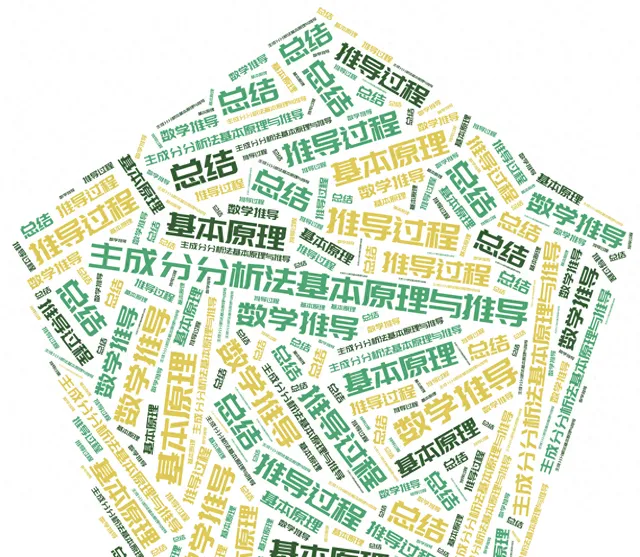
分享兴趣,传播快乐,增长见闻,留下美好!
亲爱的您,这里是LearningYard新学苑。
今天小编给您带来
【周周记:数学建模学习(28)】
欢迎您的访问!
Share interest, spread happiness, increase knowledge, leave a beautiful!
Dear, this is LearningYard New Academy.
Today, the editor brings you
"Weekly Diary: Learning Mathematical Modeling (28)"
Welcome to your visit!
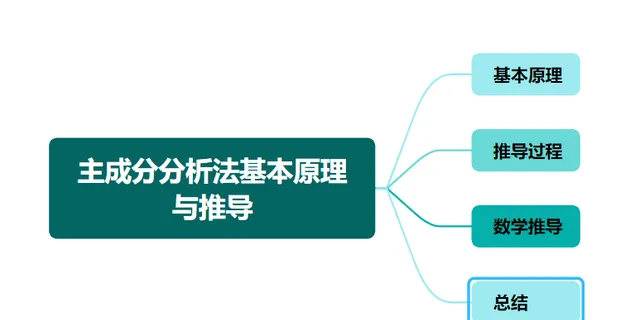
主成分分析法基本原理与推导
Basic principles and derivation of principal component analysis
一.PCA的基本原理
One. The basic principle of PCA
PCA的目标是在尽量保留原始数据集特征的前提下,降低数据的维度。这通过找到数据中方差最大的方向来实现,这些方向称为主成分。
The goal of PCA is to reduce the dimensionality of the data while preserving the characteristics of the original dataset as much as possible. This is achieved by finding the directions with the greatest variance in the data, which are called principal components.
1.数据标准化:由于特征的量纲和数值范围可能不同,首先需要对数据进行标准化处理,使得每个特征的均值为0,标准差为1。
1. Data standardization: Since the dimensions and numerical ranges of features may be different, the data needs to be normalized first, so that the mean value of each feature is 0 and the standard deviation is 1.
2. 协方差矩阵:计算标准化后数据的协方差矩阵,以确定特征之间的关系。
2. Covariance Matrix: Calculate the covariance matrix of the normalized data to determine the relationship between features.
3.特征值和特征向量:计算协方差矩阵的特征值和对应的特征向量。特征值表示特征向量方向上的方差量,而特征向量表示主成分的方向。
3. Eigenvalues and eigenvectors: Calculate the eigenvalues of the covariance matrix and the corresponding eigenvectors. The eigenvalues represent the amount of variance in the direction of the eigenvector, while the eigenvector represents the direction of the principal component.
4.选择主成分:根据特征值的大小,选择最重要的几个特征向量,这些向量是原始数据集方差最大的方向。
4. Select principal components: According to the magnitude of the eigenvalues, select the most important eigenvectors, which are the direction of the largest variance of the original data set.
5.构造新的特征空间:将原始数据投影到选定的主成分上,得到降维后的数据表示。
5. Construct a new feature space: project the original data onto the selected principal components to obtain the reduced data representation.
二.PCA的推导过程
Two. Derivation process of PCA
假设我们有 m 个 n 维的数据点,构成矩阵 X∈Rm×n。
Suppose we have m n-dimensional data points that make up the matrix X∈Rm×n.
1.中心化数据: Xcentered=X−μ
1. Centralized data: Xcentered=X−μ
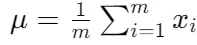
这是每个特征的均值。
There is the mean of each feature.
2.构建协方差矩阵
2. Construct a covariance matrix

这里使用 m−1作为归一化因子是为了得到无偏估计。
M−1 is used here as the normalization factor to obtain an unbiased estimate.
3.求解协方差矩阵的特征值和特征向量
3. Solve the eigenvalues and eigenvectors of the covariance matrix
求解 C 的特征值 λ λ 和对应的特征向量 v,满足: Cv=λv特征向量表示主成分的方向,特征值表示在该方向上的方差量。
Solve the eigenvalues λλ of C and the corresponding eigenvectors v, satisfying: Cv=λv eigenvectors represent the direction of the principal components, and the eigenvalues denote the variance in that direction.
4.选择主成分
4. Select principal components
按照特征值从大到小的顺序选择 k 个最大的特征值对应的特征向量,构成矩阵 P: P=[v1,v2,...,vk]。其中,vi是第 i个最大特征值对应的特征向量。
Select the eigenvectors corresponding to k largest eigenvalues in the order of eigenvalues from large to small to form the matrix P: P=[v1,v2,...,vk].where vi is the eigenvector corresponding to the i-th largest eigenvalue.
5.构造新的特征空间
5. Construct a new feature space
将原始数据投影到选定的主成分上,得到降维后的数据:
Project the original data onto the selected principal components to obtain the reduced data:
Y=XcenteredP
Y=XcenteredP
Y 是降维后的数据,其列是新的主成分,它们是线性无关的,并且包含了原始数据集中最主要的方差信息。
Y is the dimensionality reduction data, and its columns are new principal components that are linearly independent and contain the most important variance information from the original dataset.
三.数学推导
Three. Mathematical derivation
为了找到方差最大的方向,我们可以使用拉格朗日乘数法来求解最大化问题。我们希望找到方向向量 w(其中 w是单位向量,即 wTw=1),使得数据在 w w 方向上的投影的方差最大。
To find the direction with the largest variance, we can use the Lagrange multiplier method to solve the maximization problem. We want to find the direction vector w (where w is the unit vector, i.e., wTw=1) so that the variance of the projection of the data in the direction of ww is maximized.
方差的计算公式为:
The variance is calculated as:
为了最大化方差,我们需要最大化 wTCw,其中 C是协方差矩阵。
这是一个典型的特征值问题,可以通过求解以下优化问题得到:
To maximize the variance, we need to maximize wTCw, where C is the covariance matrix. This is a typical eigenvalue problem that can be obtained by solving the following optimization problem:
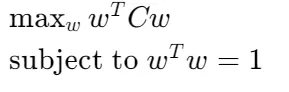
通过拉格朗日乘数法,我们可以得到 C 的特征值和特征向量。特征向量 v 就是最大化方差的单位方向向量,而对应的特征值 λ 就是最大化的方差值。
With the Lagrange multiplier method, we can get the eigenvalues and eigenvectors of C. The eigenvector v is the unit direction vector that maximizes the variance, and the corresponding eigenvalue λ is the maximized variance value.
四.总结
Four. summary
PCA通过最大化数据在新方向上的方差来找到主成分,这些主成分是原始数据集中最重要的特征。通过选择最大的几个特征值对应的特征向量,我们可以将数据投影到这些主成分上,从而实现降维。这种方法在数据预处理、特征提取和可视化中非常有用。
PCA finds the principal components by maximizing the variance of the data in the new direction, which are the most important features in the original dataset. By selecting the eigenvectors corresponding to the largest eigenvalues, we can project the data onto these principal components, thereby reducing the dimensionality. This approach is useful in data preprocessing, feature extraction, and visualization.
今天的分享就到这里了。
如果您对今天的文章有独特的看法,
欢迎给我们留言,
让我们相约明天,
祝您今天过得开心!
That's all for today's share.
If you have a unique view of today's article,
Please leave us a message,
Let's meet tomorrow,
Have a nice day!
本文由LearningYard新学苑原创,如有侵权,请联系删除。
参考资料:哔哩哔哩
翻译:Kimi